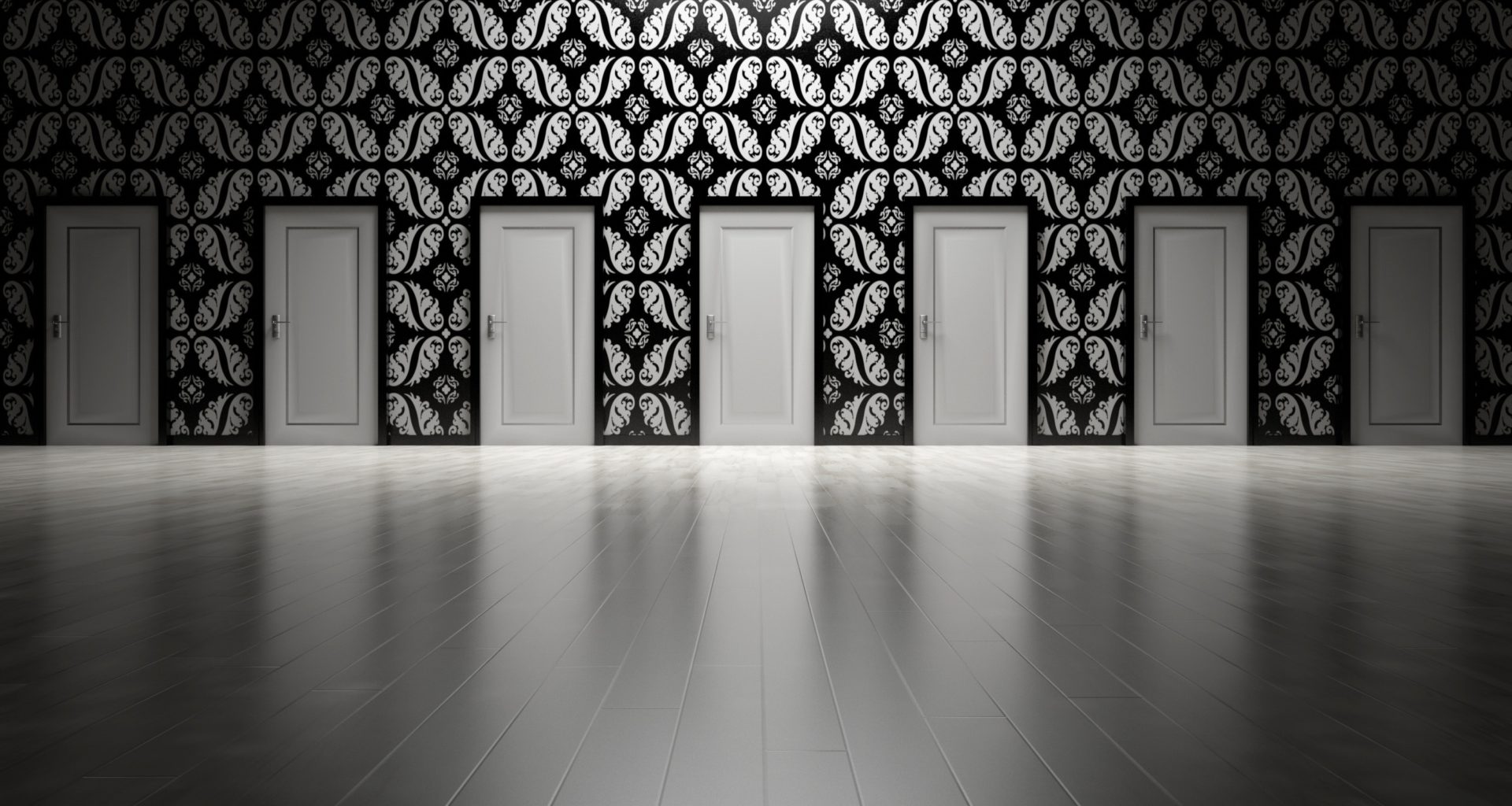
Marc Gerstein Explains Risk
Marc Gerstein, a Forbes author who was until very recently Portfolio123’s director of research (you can see his work in stock models he created like Underestimated Blue Chips), pointed out to me that when I claimed that risk and reward are not linearly correlated, I was comparing ex-ante risk and ex-post reward. I asked him to explain to me his understanding of the relationship of risk and reward, and he wrote me what follows.
It’s the clearest, most cogent defense of the Capital Asset Pricing Model (CAPM) theory of risk that I’ve read, and well worth reading. Because I want to promote on this blog a variety of ideas, not just mine, I’m publishing it below.
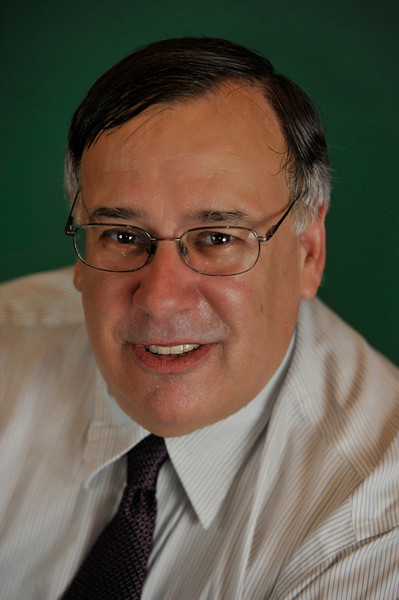
Start of Marc Gerstein‘s text.
Ex-ante (before-the-fact) expectation is just that, an expectation based on many potential outcomes that might occur. In business school we learn that to calculate expected return, identify various possible future returns, assign a probability to each (all the probabilities need to equal 1.00), and take a weighted average. That’s your ex-ante expected return. In real life, it’s done seat-of-the-pants or through some other algorithm someone may create, but ultimately it’s a statement that from all these possible outcomes, this is the one I “expect” will occur. The key is that it’s a before the fact expectation. . . .
An ex-post (after-the-fact) result is the one outcome, from all the probable outcomes, that actually occurred. That singular ex-post outcome may match the expected outcome, it may be slightly above or better, or it may have wound up being one of the more extreme outcomes. Maybe it was associated with an ex-ante probability of .65. Maybe it was associated with an ex-ante probability of 0.0005. Either way, it is just a singular observation, and it’s incumbent on anyone looking at any historical data to understand where that outcome would have fallen on the ex-ante probability continuum and why that particular outcome is the one that happened. That’s why it’s so dangerous to read too much into anything observed in the past. . . . Without understanding how much of a long shot or favorite it was and without understanding why it turned out as it did, you enter into your fight with the future completely unarmed.
Risk is something that gives you a sense of how far away from your ex-ante expectation an ex-post outcome is likely to wind up. It relates to the range of possibilities. It is not volatility. It is not standard deviation. It is not any other statistic. All of those are efforts to represent, or depict, risk. Just as an artist may do a better or worse job of depicting a likeness, so, too, may a statistician do a better or worse job of depicting risk.
Now, the key to any risk model is how effectively it captures the dynamics of this situation. The reason why CAPM was worthy of a Nobel Prize and why it is so important is that as representations of risk-reward go, it is a marvelous portrait of the ex-ante situation. Let’s go over the components one by one. . . .
Risk-free rate is the starting point. It’s based on the hard-wired human sensibility that nobody will knowingly invest in any risky asset that is expected to return less than what can be obtained with zero risk. That’s non-delusional behavior. It’s just that simple. (There is no guarantee that such an investment choice will ultimately succeed, but that is not because somebody chose to invest below the risk-free rate; it’s an unexpectedly unfavorable outcome). Next . . .
We move on to risky assets. In order to induce (bribe, if you will) anybody to invest in an asset that has any risk, you must offer an expectation that the return will be above the risk-free rate. There is no guarantee that any outcome will be successful, but you must be able to justify a before-the-fact expectation of a “premium” above the risk-free rate. This gives us the second part of CAPM, the addition of ERP (Equity Risk Premium) to RF (the risk-free rate). We’re almost home . . .
Finally, we need to consider that the equity expected return is actually a combination of individual expected returns on many equities, each of which is more or less or equally risky to the total of everything. So why, among all equities, would you choose any for which the risk is above average? You would do so only if you expect a security return to be above the average, a premium above the market return. Vice versa for lesser return and lesser risk. We need some measure of relating security return to asset class (market) return. CAPM uses Beta. In theory, it’s great, but many, including me, argue it’s not the best. Whether beta is a good or bad way to relate security risk to asset class risk, at least the theory in right on point. . . . So there we are: ER = RF + (B*ERP). That is the framework for an ex-ante assessment. Plugging in numbers in the real world is a killer (since all historical observations are plagued with the same terrible shortcoming; that we don’t know whether they reflected central or fringe outcomes from among the before-the-fact probabilities). That’s why things are hard. But the framework is the framework and it is what it is.
Therefore, when you observe that low-beta stocks have outperformed high-beta stocks over a particular period, you must ask yourself . . .
1. Was “beta” really the right measure of risk? If not, redo the assessment with another measure. If so, whether with beta or whatever else you chose . . .
2. Why of all the ex-ante probabilities did things work out such that in this outcome, lower-risk stocks outperformed? This is critical. It’s an unexpected result, so it’s important to make sense of it by understanding why before-the-fact expectations did not pan out.
3. Once you understand that, then you’re in a position to forecast, going forward, whether the aberration will continue.
Now in terms of the last several years (the Trump era), high risk has been on a tear. We had a highly pro-business leadership in Washington (almost dysfunctionally high, many would argue, e.g. the tax cut). We also had (and still have) an extreme oversupply of capital, which is manifested as extremely low-interest rates. Now, in any “market,” when supply is heavy and prices are low, this means weaker buyers are able to buy. In terms of the market, this means that lower-quality firms can get more capital more cheaply than they could in normal circumstances. So more risky companies have been seeing their stocks flourish. Small (as in “the size factor”) has an important implication in terms of higher risk very much above and beyond any conventional measure—operating leverage, internal diversification. Small risky stocks aren’t the only ones that have been flourishing; large high-risk stocks have flown as well. But if you expect your small-oriented strategies to do well going forward, you’ll have to eliminate reference to historic data and look forward to the financial markets, etc. and assess whether or not the market is likely to remain “risk on.” So far so good—in fact, small stocks have gotten better lately (IWM is not necessarily the best representation of small given its minimum cap floor and cap weighting, but many on Portfolio123 see ad hoc that small has been pretty good).
End of Marc Gerstein’s text.
For more of Marc Gerstein’s writing, I strongly suggest reading his Portfolio123 Virtual Strategy Design Class and the blog article he wrote for us last year: Beyond the Dark Ages of Factor Investing.